Genome graph methods for improved variant detection across organisms are a step change in bioinformatics practice. These relatively new but increasingly popular methods can be used to construct pangenomes (a representation of all genetic variation within a species) to facilitate better detection and understanding of complex genetic variants.
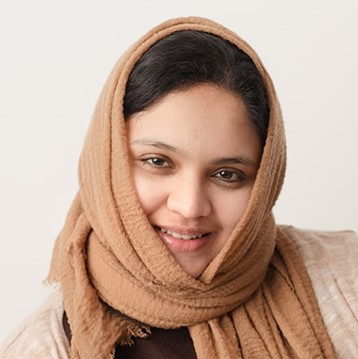
Nuzla Ismail has recently joined the Genome Graphs project as a postdoctoral fellow at the University of Otago. Her work seeks to improve the detection of structural variants in admixed populations. This work has broad applications, including disease risk prediction in human populations and breeding value estimation in agricultural stocks.
Nuzla's Genomics Aotearoa work involves:
- Bioinformatics Data Processing - working with variant graph data structures to develop workflows for turning large sequencing datasets into reproducible and robust biological findings. Most of the bioinformatics data are processed with robust Unix pipelines and data tools, followed by exploratory data analysis in the R/Python languages.
- Variant graph data structures - working mainly with vg tools (https://github.com/vgteam/vg) to construct graph genomes. Graph-based genomes have advantages over the standard linear reference genome when dealing with complex variation within a species (including genomic regions that may not be included in the linear reference).
- Simulation of population admixture, detection of complex variants and incorporation into phenotype prediction – provides a powerful method for assessing the use of genome graphs in both agricultural and human disease applications.
About Nuzla
Nuzla completed her undergraduate Bachelor of Engineering (1st Class Honours) in software engineering at Staffordshire University and a Bachelor of Science at the University of Sri Jayewardenepura, before moving to Aotearoa to complete her PhD in Information Science at the University of Otago.
Nuzla’s PhD research investigated the use of novel machine learning approaches for wildfire prediction to overcome the drawbacks of equation-based forecasting methods. Wildfires are a significant threat to people, infrastructure, and the environment. Predicting wildfire ignition is increasingly important globally because of climate change, and is needed to enable quick responses and create public awareness. However, building a predictive model with relevant features to suit different environments is challenging. Nuzla addressed this challenge by developing a proof-of-concept web-based tool to test her findings. This tool contributes to early response measures to mitigate wildfires.
Nuzla’s expertise
- Big data analytics
- Machine learning
- Software tools & technologies